The lower-post-volume people behind the software in Debian. (List of feeds.)
I keep posting about the importance of functions inside of deep neural networks being sublinear but haven’t given an exact definition of that before. It’s sublinearity in the computer science asymptotic sense. The Taylor expansion should not only have a linear bound but either going to zero or at least have the positive and negative directions go to different asymptotics. If the function is defined by different formulas in different sections that criterion should apply to all of them.1
With that out the the way here’s how common activation functions look with a new suggestion at the bottom.
This is as nonlinear as you can get a monotonic function sublinear function to be and is trivial to compute. The one big problem is that it has that kink at 0. Is it too much to ask for a function to be continuously differentiable?
It’s okay to have insecurities about some areas being completely occluded and hence stop responding to training but if it’s that much of a problem for you you don’t have what it takes to work with DNNs and should go back to playing with linear functions.
GELU
We get it, you got rid of that kink, but the requirements specified a function which is monotonic, can you not read? Also maybe don’t use functions which are so obscure that I can’t figure out how to enter them into Wolfram Alpha.
Thank you for you following the requirements and there’s some argument to using Softmax here since you’re probably using it elsewhere anyway. But it does seem to take a large area to smooth that kink out and never quite gets to exactly RELU in either direction.
RELU with Sinusoidal Smoothing (RELUSS)
This is my new idea. Not only is the kink completely smoothed out, it’s done with a simple quick to calculate function which meets the requirements and reverts completely to RELU outside of that area.
Don’t ask about x*sin(x)
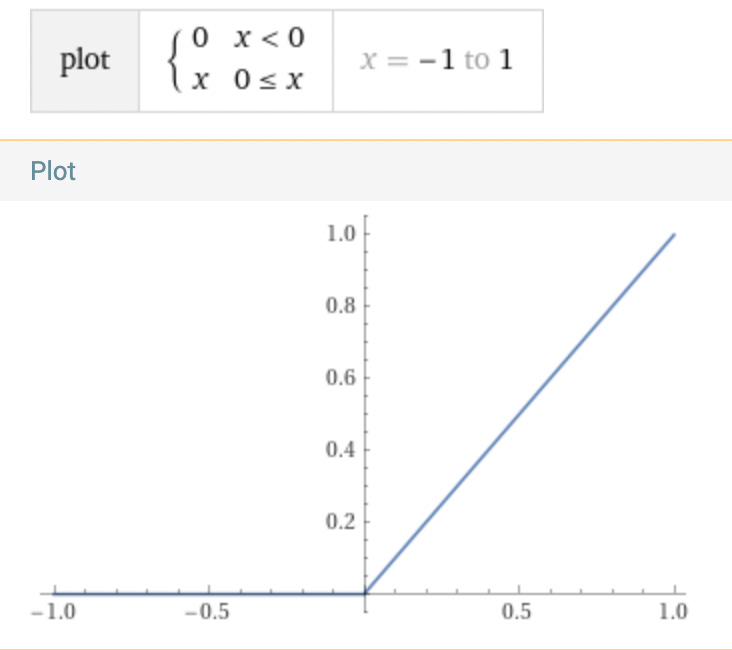
Over the last months I've started looking into a few of the papercuts that affects graphics tablet users in GNOME. So now that most of those have gone in, let's see what has happened:
Calibration fixes and improvements (GNOME 47)
The calibration code, a descendent of the old xinput_calibrator tool was in a pretty rough shape and didn't work particularly well. That's now fixed and I've made the calibrator a little bit easier to use too. Previously the timeout was quite short which made calibration quite stressfull, that timeout is now per target rather than to complete the whole calibration process. Likewise, the calibration targets now accept larger variations - something probably not needed for real use-cases (you want the calibration to be exact) but it certainly makes testing easier since clicking near the target is good enough.
The other feature added was to allow calibration even when the tablet is manually mapped to a monitor. Previously this only worked in the "auto" configuration but some tablets don't correctly map to the right screen and lost calibration abilities. That's fixed now too.
A picture says a thousand words, except in this case where the screenshot provides no value whatsoever. But here you have it anyway.
Generic tablet fallback (GNOME 47)
Traditionally, GNOME would rely on libwacom to get some information about tablets so it could present users with the right configuration options. The drawback was that a tablet not recognised by libwacom didn't exist in GNOME Settings - and there was no immediately obvious way of fixing this, the panel either didn't show up or (with multiple tablets) the unrecognised one was missing. The tablet worked (because the kernel and libinput didn't require libwacom) but it just couldn't be configured.
libwacom 2.11 changed the default fallback tablet to be a built-in one since this is now the most common unsupported tablet we see. Together with the new fallback handling in GNOME settings this means that any unsupported tablet is treated as a generic built-in tablet and provides the basic configuration options for those (Map to Monitor, Calibrate, assigning stylus buttons). The tablet should still be added to libwacom but at least it's no longer a requirement for configuration. Plus there's now a link to the GNOME Help to explain things. Below is a screenshot on how this looks like (after modifying my libwacom to no longer recognise the tablet, poor Intuos).
Monitor mapping names (GNOME 47)
For historical reasons, the names of the display in the GNOME Settings Display configuration differed from the one used by the Wacom panel. Not ideal and that bit is now fixed with the Wacom panel listing the name of the monitor and the connector name if multiple monitors share the same name. You get the best value out of this if you have a monitor vendor with short names. (This is not a purchase recommendation).
Highlighted SVGs (GNOME 46)
If you're an avid tablet user, you may have multiple stylus tools - but it's also likely that you have multiple tools of the same type which makes differentiating them in the GUI hard. Which is why they're highlighted now - if you bring the tool into proximity, the matching image is highlighted to make it easier to know which stylus you're about to configure. Oh, and in the process we added a new SVG for AES styli too to make the picture look more like the actual physical tool. The <blink> tag may no longer be cool but at least we can disco our way through the stylus configuration now.
More Pressure Curves (GNOME 46)
GNOME Settings historically presents a slider from "Soft" to "Firm" to adjust the feel of the tablet tip (which influences the pressure values sent to the application). Behind the scenes this was converted into a set of 7 fixed curves but thanks to a old mutter bug those curves only covered a small amount of the possible range. This is now fixed so you can really go from pencil-hard to jelly-soft and the slider now controls an almost-continous range instead of just 7 curves. Behold, a picture of slidery goodness:
Miscellaneous fixes
And of course a bunch of miscellaneous fixes. Things that I quickly found were support for Alt in the tablet pad keymappings, fixing of erroneous backwards movement when wrapping around on the ring, a long-standing stylus button mismatch, better stylus naming and a rather odd fix causing configuration issues if the eraser was the first tool ever to be brought into proximity.
There are a few more things in the pipe but I figured this is enough to write a blog post so I no longer have to remember to write a blog post about all this.
SwiftNavigation
To celebrate that RealityKit's is coming to MacOS, iOS and iPadOS and is no longer limited to VisionOS, I am releasing SwiftNavigation for RealityKit.
Last year, as I was building a game for VisionPro, I wanted the 3D characters I placed in the world to navigate the world, go from one point to another, avoid obstacles and have those 3D characters avoid each other.
Almost every game engine in the world uses the C++ library RecastNavigation library to do this - Unity, Unreal and Godot all use it.
SwiftNavigation was born: Both a Swift wrapper to the underlying C++ library which leverages extensively Swift's C++ interoperability capabilities and it directly integrates into the RealityKit entity system.
This library is magical, you create a navigation mesh from the world that you capture and then you can query it for paths to navigate from one point to another or you can create a crowd controller that will automatically move your objects.
Until I have the time to write full tutorials, your best bet is to look at the example project that uses it.
My grandmother used to make a recipe from an old newspaper clipping. After decades the original clipping started to crumble so she replaced it with a new clipping when the newspaper re-ran the recipe. I struggled but eventually succeeded in making a recipe which matched my childhood memories. Sadly my childhood memories were romanticized and my grandmother’s original recipe didn’t make the pancakes stay floofed after they were done cooling off, but I hope you enjoy this improved version.
These pancakes rise by water under the batter turning into steam, so to keep the pan from getting cooled off by the batter it’s important to cook them in an iron skillet which has been given time to heat all the way through.
3 eggs
70 grams flour
120 grams milk
1 gram nutmeg
1 gram mint oil
Pinch of salt
6 grams powdered sugar
3 grams Lemon juice powder
20 grams Ghee
Preheat the oven with a skillet inside to 400 degrees. Leave it in for 15 more minutes after preheating. Mix together eggs, flour, milk, nutmeg, mint oil, and salt and beat thoroughly. When the oven is heated add ghee to the pan and put it back in to melt (about 2 minutes). After it’s done melting, pour batter on top. Bake for 20 minutes. Thoroughly mix powdered sugar and lemon juice powder and put it in a dusting wand. Sift completely over top.
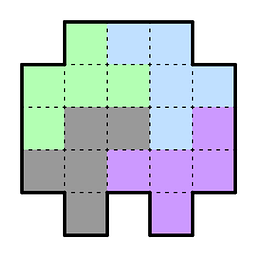
Back in the day when presumably at least someone was young, the venerable xsetwacom tool was commonly used to configure wacom tablets devices on Xorg [1]. This tool is going dodo in Wayland because, well, a tool that is specific to an X input driver kinda stops working when said X input driver is no longer being used. Such is technology, let's go back to sheep farming.
There's nothing hugely special about xsetwacom, it's effectively identical to the xinput commandline tool except for the CLI that guides you towards the various wacom driver-specific properties and knows the right magic values to set. Like xinput, xsetwacom has one big peculiarity: it is a fire-and-forget tool and nothing is persistent - unplugging the device or logging out would vanish the current value without so much as a "poof" noise [2].
If also somewhat clashes with GNOME (or any DE, really). GNOME configuration works so that GNOME Settings (gnome-control-center) and GNOME Tweaks write the various values to the gsettings. mutter [3] picks up changes to those values and in response toggles the X driver properties (or in Wayland the libinput context). xsetwacom short-cuts that process by writing directly to the driver but properties are "last one wins" so there were plenty of use-cases over the years where changes by xsetwacom were overwritten.
Anyway, there are plenty of use-cases where xsetwacom is actually quite useful, in particular where tablet behaviour needs to be scripted, e.g. switching between pressure curves at the press of a button or key. But xsetwacom cannot work under Wayland because a) the xf86-input-wacom driver is no longer in use, b) only the compositor (i.e. mutter) has access to the libinput context (and some behaviours are now implemented in the compositor anyway) and c) we're constantly trying to think of new ways to make life worse for angry commenters on the internets. So if xsetwacom cannot work, what can we do?
Well, most configurations possible with xsetwacom are actually available in GNOME. So let's make those available to a commandline utility! And voila, I present to you gsetwacom, a commandline utility to toggle the various tablet settings under GNOME:
$ gsetwacom list-devices devices: - name: "HUION Huion Tablet_H641P Pen" usbid: "256C:0066" - name: "Wacom Intuos Pro M Pen" usbid: "056A:0357" $ gsetwacom tablet "056A:0357" set-left-handed true $ gsetwacom tablet "056A:0357" set-button-action A keybinding "<Control><Alt>t" $ gsetwacom tablet "056A:0357" map-to-monitor --connector DP-1
Just like xsetwacom was effectively identical to xinput but with a domain-specific CLI, gsetwacom is effectively identical to the gsettings tool but with a domain-specific CLI. gsetwacom is not intended to be a drop-in replacement for xsetwacom, the CLI is very different. That's mostly on purpose because I don't want to have to chase bug-for-bug compatibility for something that is very different after all.
I almost spent more time writing this blog post than on the implementation so it's still a bit rough. Also, (partially) due to how relocatable schemas work error checking is virtually nonexistent - if you want to configure Button 16 on your 2-button tablet device you can do that. Just don't expect 14 new buttons to magically sprout from your tablet. This could all be worked around with e.g. libwacom integration but right now I'm too lazy for that [4]
Oh, and because gsetwacom writes the gsettings configuration it is persistent, GNOME Settings will pick up those values and they'll be re-applied by mutter after unplug. And because mutter-on-Xorg still works, gsetwacom will work the same under Xorg. It'll also work under the GNOME derivatives as long as they use the same gsettings schemas and keys.
Le utilitaire est mort, vive le utilitaire!
[1] The git log claims libwacom was originally written in 2009. By me. That was a surprise...
[2] Though if you have the same speakers as I do you at least get a loud "pop" sound whenever you log in/out and the speaker gets woken up
[3] It used to be gnome-settings-daemon but with mutter now controlling the libinput context this all moved to mutter
[4] Especially because I don't want to write Python bindings for libwacom right now
As I’ve mentioned previously if you want eventually consistent version control, meaning whatever order you merge things together has no impact on the final result, you not only need to have a very history aware merging algorithm, you also need canonical ordering of the lines. This cleanly dodges around the biggest issue in version control, which is what should you do when one person merges AXB and AYB as AXYB and another person merges them together as AYXB and then you try to merge both of those together. None of the available options are good, so you have to keep it from ever happening in the first place. Both people need to be shown AXYB as the order of lines in the merge conflict (or the other order as long as it’s consistent) and that way if either of them decided to change it to AYXB then that was a proactive change made afterwards and is not only a winner of the later meta-merge conflict, there isn’t even a conflict at all, it merges cleanly.
This flies in the face of how UX normally works on merge conflicts, which orders the conflicting regions by whether they’re ‘local’ or ‘remote’. How to do order better is an involved subject which I’ve covered thoroughly in older posts and won’t rehash here, but conflict UX I want to talk about more. Since the order of lines and whether they should be included if everything is smashed together blindly is assumed to be handled, that creates a question of how to detect and present conflicts. What’s going to be needed is a way of marking particular lines as conflicts and figuring out what should be marked. There should be some format of special lines similar to the conflict markers people are already familiar with as a way of presenting them to users in files. That format should include a way of saying which of the two sides individual lines came from.
The general idea is to determine ‘which side each line came from’ and if two lines whose ancestry are different are ‘too close together’ then they’re both marked as being in conflict. If successive lines have the same ancestry then if one of them is in conflict it taints the others. The simplest approach is that a single line of code which is present on both sides ends regions of conflict. Arguably it should be more than one line to declare peace, or that empty or whitespace only lines shouldn’t count towards it. I’m going to assume the simplest approach for a proof of concept.
An important case is when Alice adds a line to a function and Bob deletes the entire function. Obviously that should somehow be presented as a conflict but deleted lines are crucial to it. For that reason there needs to be some way of showing deleted lines in the conflict, definitely with proper annotations around them and possibly with the individual deleted lines commented out.
To detect conflicts each line is marked as ‘peaceful’, ‘skip’, ‘Alice won’, ‘Bob won’ or ‘both won‘. Once all lines are marked then the ones which are marked skip are, well, skipped. Other lines which border lines with a different marking which is not peaceful are marked as in conflict. Finally tainting is spread to neighboring lines which have the same state. Deleted lines are only presented to the user if they’re in conflict.
What to do in each case is best presented as a laundry list, so here goes. Each case is final-Alice-Bob.
missing missing missing: skip
missing missing present: Alice
missing present missing: Bob
missing present present: both (this is an unusual case but it can happen)
present missing missing: both (similar to the previous case)
present missing present: Bob
present present missing: Alice
present present present: peaceful
That seems to handle all the edge cases properly and covers the last of the theoretical details I needed to work out.
When a user resolves a conflict and does a commit it should first throw an error if conflict markers weren’t removed, then should assume the user edited the clean merge they would have seen if each line were presented verbatim without checking for conflicts. When doing a diff between the complete weave and the user’s final file version it should probably more heavily weight lines which were present than lines which were deleted but I’m not sure what the best way of doing that is and will probably make a prototype which doesn’t have any such heuristic.
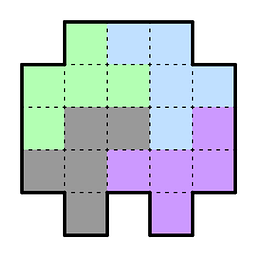
PLA is a great 3D printing material with one major flaw. It’s the stiffest of available materials, not toxic, cheap, and prints easily. The main downside of it is that it melts at an annoyingly low temperature. It would be nice to have some material which is like PLA in all characteristics but has a higher melting temperature. It turns out that such a material exists and it is… PLA.
That last statement requires some explaining. The distinction is whether PLA is annealed or not. Annealing is a process where a material is brought up to a high temperature and then very slowly cooled down, causing it to be more crystalline (or at least lower energy) at the molecular level and thus stronger/tougher/having a higher melting point. PLA the material does this very well but if you apply the process to 3d printed parts they warp because internal stresses within the parts get released. It’s like the objects are made out of frozen rubber bands which were stretched out as the filament was layed down and heating it up allows them to spring shut.
The causes of this problem are that the filament wasn’t made hot enough when it was extruded and wasn’t cooled down slowly enough afterwards. The straightforward way of fixing this would be to do exactly that: make the filament so hot it’s a liquid when it comes out, then cool everything down slowly afterwards. That would require some kind of soluble support material which is solid at those high temperatures, printing everything at 100% fill because it’s a liquid, and keeping the entire 3D printer at those high temperatures. While this approach may work it’s unlikely the printer itself would still be cheap and reliable with all that literally getting cooked while it’s running.
A more practical approach may be to invent a PLA blend which anneals better. If PLA is interleaved with another material which forms a matrix around it, maybe that other material could melt at a much higher temperature, meaning it’s still frozen at the temperature PLA needs to be heated to to get it to anneal, so doing the annealing process post-printing wouldn’t cause the item to warp. The obvious candidate for this is carbon fiber. Maybe you could make carbon fiber PLA with massively more carbon fiber than anything currently available, to the point where the melting point is dramatically increased, then print using that and anneal later by taking the parts back up to the melting point of PLA but not the melting point of the combined material. Whether carbon fiber specifically or anything in general can get PLA to behave that way I don’t know, and obviously a brass nozzle couldn’t handle that material, but maybe some experimentation could result in a new type of filament which could make very high quality parts quickly and easily.
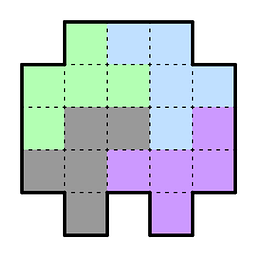
TLDR: Thanks to José Exposito, libwacom 2.12 will support all [1] Huion and Gaomon devices when running on a 6.10 kernel.
libwacom, now almost 13 years old, is a C library that provides a bunch of static information about graphics tablets that is not otherwise available by looking at the kernel device. Basically, it's a set of APIs in the form of libwacom_get_num_buttons and so on. This is used by various components to be more precise about initializing devices, even though libwacom itself has no effect on whether the device works. It's only a library for historical reasons [2], if I were to rewrite it today, I'd probably ship libwacom as a set of static json or XML files with a specific schema.
Here are a few examples on how this information is used: libinput uses libwacom to query information about tablet tools.The kernel event node always supports tilt but the individual tool that is currently in proximity may not. libinput can get the tool ID from the kernel, query libwacom and then initialize the tool struct correctly so the compositor and Wayland clients will get the right information. GNOME Settings uses libwacom's information to e.g. detect if a tablet is built-in or an external display (to show you the "Map to Monitor" button or not, if builtin), GNOME's mutter uses the SVGs provided by libwacom to show you an OSD where you can assign keystrokes to the buttons. All these features require that the tablet is supported by libwacom.
Huion and Gamon devices [3] were not well supported by libwacom because they re-use USB ids, i.e. different tablets from seemingly different manufacturers have the same vendor and product ID. This is understandable, the 16-bit product id only allows for 65535 different devices and if you're a company that thinks about more than just the current quarterly earnings you realise that if you release a few devices every year (let's say 5-7), you may run out of product IDs in about 10000 years. Need to think ahead! So between the 140 Huion and Gaomon devices we now have in libwacom I only counted 4 different USB ids. Nine years ago we added name matching too to work around this (i.e. the vid/pid/name combo must match) but, lo and behold, we may run out of unique strings before the heat death of the universe so device names are re-used too! [4] Since we had no other information available to userspace this meant that if you plugged in e.g. a Gaomon M106 and it was detected as S620 and given wrong button numbers, a wrong SVG, etc.
A while ago José got himself a tablet and started contributing to DIGIMEND (and upstreaming a bunch of things). At some point we realised that the kernel actually had the information we needed: the firmware version string from the tablet which conveniently gave us the tablet model too. With this kernel patch scheduled for 6.10 this is now exported as the uniq property (HID_UNIQ in the uevent) and that means it's available to userspace. After a bit of rework in libwacom we can now match on the trifecta of vid/pid/uniq or the quadrella of vid/pid/name/uniq. So hooray, for the first time we can actually detect Huion and Gaomon devices correctly.
The second thing Jose did was to extract all model names from the .deb packages Huion and Gaomon provide and auto-generate all libwacom descriptions for all supported devices. Which meant, in one pull request we added around 130 devices. Nice!
As said above, this requires the future kernel 6.10 but you can apply the patches to your current kernel if you want. If you do have one of the newly added devices, please verify the .tablet file for your device and let us know so we can remove the "this is autogenerated" warnings and fix any issues with the file. Some of the new files may now take precedence over the old hand-added ones so over time we'll likely have to merge them. But meanwhile, for a brief moment in time, things may actually work.
[1] fsvo of all but should be all current and past ones provided they were supported by Huions driver
[2] anecdote: in 2011 Jason Gerecke from Wacom and I sat down to and decided on a generic tablet handling library independent of the xf86-input-wacom driver. libwacom was supposed to be that library but it never turned into more than a static description library, libinput is now what our original libwacom idea was.
[3] and XP Pen and UCLogic but we don't yet have a fix for those at the time of writing
[4] names like "HUION PenTablet Pen"...
Car fobs have a security problem. If you’ve parked your car in front of your house someone can relay messages between your key fob and your car, get your car to unlock, get in, and drive off.
This attack is possible because of a sensor problem: The fob and car rely on the strength of the signal between them to sense how far away they are from each other, and that strength can be boosted by an attacker. Thankfully there’s an improved method of sensing distance which is long overdue for being the standard technique, which is to rely on the speed of light. If the fob and car are close enough the round trip time between the two will be low, and if they’re too far away then an intermediary echoing messages can’t reduce the round trip time, only increase it. Thank you absolute speed of light.
As compelling as this is in principle implementing it can be tricky because the processing on the end point needs to be faster than the round trip time. Light goes about a foot in a nanosecond, so you want your total processing time down to a few nanoseconds at the most. This is plenty of time for hardware to do something, but between dodgy and impossible to do any significant amount of computation. But there’s a silly trick for fixing the problem.
Any protocol between the car and fob will end with one final message sent by the fob. To make it round trip secure the fab instead signals to the car that it’s ready to send the final message at which point the car generates a random one time pad and sends it back to the fob, at which point the fob xors the final message with the pad and sends that as the final message. The car can then xor again to get the real final message, authenticate it however is required of the underlying protocol, and if the round trip time on the final message was low enough open up. This allows the fob to calculate its final message at its leisure then load it into something at the hardware level which does xor-and-pong. A similar hardware level thing on the car side can be told to generate a ping with one time pad, then return back the pong message along with a round trip time to receive it. That way all the cryptography can be done at your leisure in a regular programming environment and the low latency stuff is handled by hardware. If you want to be fancy when making the hardware you can even support an identifying code which needs to match on the sending and receiving sides so messages don’t interfere with each other.
Distance detection used on point of sale devices should also work this way. That would have the benefit you wouldn’t have to smush the paying device’s face right into the point of sale machine just to get a reading. The protocol should be a little different for that because in a real payment protocol the paying device should authenticate the point of sale machine rather than the other way around. But the credit card approach does things backwards, and it seems likely that if hardware capability of this sort of thing is built into phones it will be the wrong side.
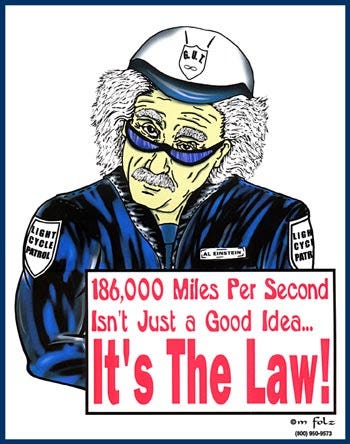
In my last post (which this post is a superior rehashing of after thinking things over more) I talked about ‘chaos’ which seemed to leave some people confused as to what that meant. Despite being a buzzword which is thrown around in pop science a lot chaos is a real mathematical term with a very pedestrian definition, which is sensitive dependence on initial conditions. It’s a simultaneously banal and profound observation to point out that neural networks as we know them today are critically dependent on not having sensitive dependence on initial conditions in order for back propagation to work properly.
It makes sense to refer to these as ‘sublinear’ functions, a subset of all nonlinear functions. It feels like the details of how sublinear functions are trained don’t really matter all that much. More data, training, and bigger models will get you better results but still suffer from some inherent limitations. To get out of their known weaknesses you have to somehow include superlinear functions, and apply a number of them stacked deep to get the potential for chaotic behavior. LLMs happen to need to throw in a superlinear function because picking out a word among possibilities is inherently superlinear. To maximize an LLMs performance (or at least its superlinearity) you should make it output a buffer of as many relevant words as possible in between the question and where it gives an answer, to give it a chance to ‘think out loud’. Instead of asking it to simply give an answer, ask it to give several different answers, then make give arguments for and against each of those, then give rebuttals to those arguments, then write several new answers taking all of that into account, repeat the exercise of arguments for and against with rebuttals, and finally pick out which if its answers is best. This is very much in line with the already known practical ways of getting better answers out of LLMs and likely to work well. It also seems like a very human process which raises the question of whether the human brain also consists of a lot of sublinear regions with superlinear controllers. We have no idea.
What got me digging into the workings of LLMs was that I got wind that they use dot products in a place and wondered whether the spatial clustering I’ve been working on could be applied. It turns out it can’t, because it requires gradient descent, and gradient descent on top of being expensive is also extremely chaotic. But there is a very simple thing which is sublinear which can be tried: Apply RELU/GRELU to the key and query vectors (or maybe just one of them, a few experiments can be done) before taking their dot product. You might call this the ‘pay attention to the man behind the curtain’ heuristic, because it works with the intuition that there can be reasons why you should pay special attention to something but not many reasons why you shouldn’t.
For image generation the main thing you need is some kind of superlinear function applied before iterations of using a neural network to make the image better. With RGB values expressed as being between 0 and 1 it appears that the best function is to square everything. The reasoning here is that you want the second derivative to be as much as possible everywhere and evenly spread out while keeping the function monotonic and within the defined range. The math on that yields two quadratics, x^2 and its cousin -x^2+2x. There are a few reasons why this logical conclusion sounds insane. First there are two functions for no apparent reason. Maybe it makes sense to alternate between them? Less silly is that it’s a weird bit of magic pixie dust, but then adding random noise is also magic pixie dust but seems completely legit. It also does something cognitively significant, but then it’s common for humans to make a faint version of an image and draw over it and this seems very reminiscent of that. The point of making the image faint is to be information losing, and simply multiplying values isn’t information losing within the class of sublinear functions while square is because if you do it enough times everything gets rounded down to zero.
Frustratingly image classification isn’t iterated and so doesn’t have an obvious place to throw in superlinear functions. Maybe it could be based off having a witness to a particular classification and have that be iteratively improved. Intuitively a witness traces over the part of the image which justifies the classification, sort of like circling the picture of Waldo. But image classification doesn’t use witnesses and it isn’t obvious how to make them do that so a new idea is needed.
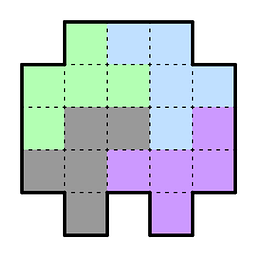
For the last few months, Benjamin Tissoires and I have been working on and polishing a little tool called udev-hid-bpf [1]. This is the scaffolding required quickly and easily write, test and eventually fix your HID input devices (mouse, keyboard, etc.) via a BPF program instead of a full-blown custom kernel driver or a semi-full-blown kernel patch. To understand how it works, you need to know two things: HID and BPF [2].
Why BPF for HID?
HID is the Human Interface Device standard and the most common way input devices communicate with the host (HID over USB, HID over Bluetooth, etc.). It has two core components: the "report descriptor" and "reports", both of which are byte arrays. The report descriptor is a fixed burnt-in-ROM byte array that (in rather convoluted terms) tells us what we'll find in the reports. Things like "bits 16 through to 24 is the delta x coordinate" or "bit 5 is the binary button state for button 3 in degrees celcius". The reports themselves are sent at (usually) regular intervals and contain the data in the described format, as the devices perceives reality. If you're interested in more details, see Understanding HID report descriptors.
BPF or more correctly eBPF is a Linux kernel technology to write programs in a subset of C, compile it and load it into the kernel. The magic thing here is that the kernel will verify it, so once loaded, the program is "safe". And because it's safe it can be run in kernel space which means it's fast. eBPF was originally written for network packet filters but as of kernel v6.3 and thanks to Benjamin, we have BPF in the HID subsystem. HID actually lends itself really well to BPF because, well, we have a byte array and to fix our devices we need to do complicated things like "toggle that bit to zero" or "swap those two values".
If we want to fix our devices we usually need to do one of two things: fix the report descriptor to enable/disable/change some of the values the device pretends to support. For example, we can say we support 5 buttons instead of the supposed 8. Or we need to fix the report by e.g. inverting the y value for the device. This can be done in a custom kernel driver but a HID BPF program is quite a lot more convenient.
HID-BPF programs
For illustration purposes, here's the example program to flip the y coordinate. HID BPF programs are usually device specific, we need to know that the e.g. the y coordinate is 16 bits and sits in bytes 3 and 4 (little endian):
SEC("fmod_ret/hid_bpf_device_event") int BPF_PROG(hid_y_event, struct hid_bpf_ctx *hctx) { s16 y; __u8 *data = hid_bpf_get_data(hctx, 0 /* offset */, 9 /* size */); if (!data) return 0; /* EPERM check */ y = data[3] | (data[4] << 8); y = -y; data[3] = y & 0xFF; data[4] = (y >> 8) & 0xFF; return 0; }That's it. HID-BPF is invoked before the kernel handles the HID report/report descriptor so to the kernel the modified report looks as if it came from the device.
As said above, this is device specific because where the coordinates is in the report depends on the device (the report descriptor will tell us). In this example we want to ensure the BPF program is only loaded for our device (vid/pid of 04d9/a09f), and for extra safety we also double-check that the report descriptor matches.
// The bpf.o will only be loaded for devices in this list HID_BPF_CONFIG( HID_DEVICE(BUS_USB, HID_GROUP_GENERIC, 0x04D9, 0xA09F) ); SEC("syscall") int probe(struct hid_bpf_probe_args *ctx) { /* * The device exports 3 interfaces. * The mouse interface has a report descriptor of length 71. * So if report descriptor size is not 71, mark as -EINVAL */ ctx->retval = ctx->rdesc_size != 71; if (ctx->retval) ctx->retval = -EINVAL; return 0; }Obviously the check in probe() can be as complicated as you want.
This is pretty much it, the full working program only has a few extra includes and boilerplate. So it mostly comes down to compiling and running it, and this is where udev-hid-bpf comes in.
udev-hid-bpf as loader
udev-hid-bpf is a tool to make the development and testing of HID BPF programs simple, and collect HID BPF programs. You basically run meson compile and meson install and voila, whatever BPF program applies to your devices will be auto-loaded next time you plug those in. If you just want to test a single bpf.o file you can udev-hid-bpf install /path/to/foo.bpf.o and it will install the required udev rule for it to get loaded whenever the device is plugged in. If you don't know how to compile, you can grab a tarball from our CI and test the pre-compiled bpf.o. Hooray, even simpler.
udev-hid-bpf is written in Rust but you don't need to know Rust, it's just the scaffolding. The BPF programs are all in C. Rust just gives us a relatively easy way to provide a static binary that will work on most tester's machines.
The documentation for udev-hid-bpf is here. So if you have a device that needs a hardware quirk or just has an annoying behaviour that you always wanted to fix, well, now's the time. Fixing your device has never been easier! [3].
[1] Yes, the name is meh but you're welcome to come up with a better one and go back in time to suggest it a few months ago.
[2] Because I'm lazy the terms eBPF and BPF will be used interchangeably in this article. Because the difference doesn't really matter in this context, it's all eBPF anyway but nobody has the time to type that extra "e".
[3] Citation needed
I’ve been looking into the inner workings of neural networks and have some thoughts about them. First and foremost the technique of back propagation working at all is truly miraculous. This isn’t an accident of course, the functions used are painstakingly picked out so that this amazing back propagation can work. This puts a limitation on them that they have to be non-chaotic. It appears to be that non-chaotic functions as a group are something of a plateau, sort of like how linear functions are a plateau, but with a much harder to characterize set of capabilities and weaknesses. But one of them is that they’re inherently very easy to attack using white box techniques and the obvious defenses against those attacks, very much including the ones I’ve proposed before, are unlikely to work. Harumph.
To a first approximation the way to get deep neural networks to perform better is to fully embrace their non-chaotic nature. The most striking example of this is in LLMs whose big advance was to dispense with recursive state and just use attention. The problem with recursiveness isn’t that it’s less capable. It’s trivially more general so at first everyone naively assumed it was better. The problem is that recursiveness leads to exponentialness which leads to chaos and back propagation not working. This is a deep and insidious limitation, and trying to attack it head on tends to simply fail.
At this point you’re probably expecting me to give one weird trick which fixes this problem, and I will, but be forewarned that this just barely gets outside of non-chaos. It isn’t about to lead to AGI or anything.
The trick is to apply the non-chaotic function iteratively with some kind of potentially chaos-inducing modification step thrown in between. Given how often chaos happens normally this is a low bar. The functions within deep neural networks are painstakingly chosen so that their second derivative is working to keep their first derivative under control at all times. All the chaos inducing functions have to do is let their second derivative’s freak flag fly.
LLMs do this by accident because they pick a word at a time and the act of committing to a next word is inherently chaotic. But they have a limitation that their chaoticism only comes out a little bit at a time so they have to think out loud to get anywhere. LLM performance may be improved by letting it run and once in a while interjecting that now is the time to put together a summary of all the points and themes currently in play and give the points and themes it intends to use in the upcoming section before it continues. Then in the end elide the notes. In addition to letting it think out loud this also hacks around context window problems because information from earlier can get carried forward in the summaries. This is very much in the vein of standard issue LLM hackery and has a fairly high chance of working. It also may be useful writing advice to humans whose brains happen to be made out of neural networks.
Applying the same approach to image generation requires repeatedly iterating on an image to improve it with each stage. Diffusion sort of works this way, although it works off the intuition that further details are getting filled in each time. This analysis seems to indicate that the real benefit is that making a pixellated image is doing something chaotic, on the same order of crudeness as forcing the picking out of a next word from an LLM. Instead it may better to make each step work on a detailed image and apply something chaos-inducing in between. It may be that adding gaussian noise works, but as ridiculous as it sounds in principle doing color enhancement using a cubic function should work far better. I have no idea if this idea actually works. It sounds simultaneously on very sound mathematical footing and completely insane.
Annoyingly I don’t see a way of doing image classification as an iterative process with something chaos-inducing in between steps. Maybe there’s another silly trick there which would be able to make the white box attacks not work so well.
Side note: It seems like there should be a better term for a function which is ‘not non-chaotic’. They don’t have to be at all chaotic themselves, just contain the seeds of chaos. Even quadratic functions fit the bill, although cubic ones are a bit easier to throw in because they can be monotonic.
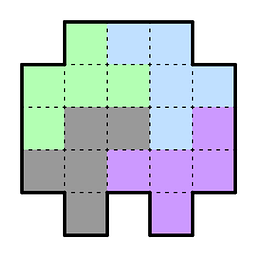